TrueFoundry makes it really easy to deploy applications on Kubernetes clusters in your own cloud provider account. It does so by abstracting out the infrastructure components for data scientists and developers while enforcing the best practices from security, infrastructure, and cost optimization perspectives.
The key motivations behind the current architecture of TrueFoundry are:
- Data shouldn't leave your cloud/on-prem account: Machine Learning usually involves interacting with a lot of data. If data flows out of your cloud account (VPC), there is a risk of data security getting compromized and we also end up incurring data egress/ingress costs. This is why TrueFoundry was designed from ground up to keep data and compute both inside your own environment.
- ML inherits the SRE principles within your organization: Companies usually implement the entire deployment, monitoring and alerting stacks for deploying their software microservices. We wanted ML to inherit the same practices and not go a parallel infrastructure setup route. This makes it easy for infrastructure and SRE teams to enforce the security and cost optimization best practices throughout the entire organization.
- Cloud Native: TrueFoundry is built on Kubernetes and hence cloud-native by design. However, even though Kubernetes is cloud-native, there are a lot of intricate differences between the Kubernetes distributions of AWS(EKS), GCP (GKE) and Azure (AKS). A few examples of these differences are:
GKE Autopilot enforces having same values for requests and limits for resources, while AKS, EKS and GKE Standard do not.
EKS and GKE have an option for auto-node provisioning, while AKS doesn't provide a way for that.
Node provisioning time is quite high for AKS, which leads to very slow autoscaling behaviour.
Being cloud-native allows us to have access to the differnet hardware provided by different cloud providers specially in case of GPUs.
4. Integrate rather than reinvent: TrueFoundry integrates with most of the commonly used systems instead of reinventing the wheel. This philosophy drives a lot of our architecture decisions. It does sometimes make the journey harder for us since its not always easy to build integrations where solid APIs are not available - but we do the hard work of building those APIs and interfaces so that our users don't need to learn yet another tool.
ML Stack for fast iteration and impact
Machine Learning requires a complicated stack to be setup for datascientists to experiment and deliver rapidly.
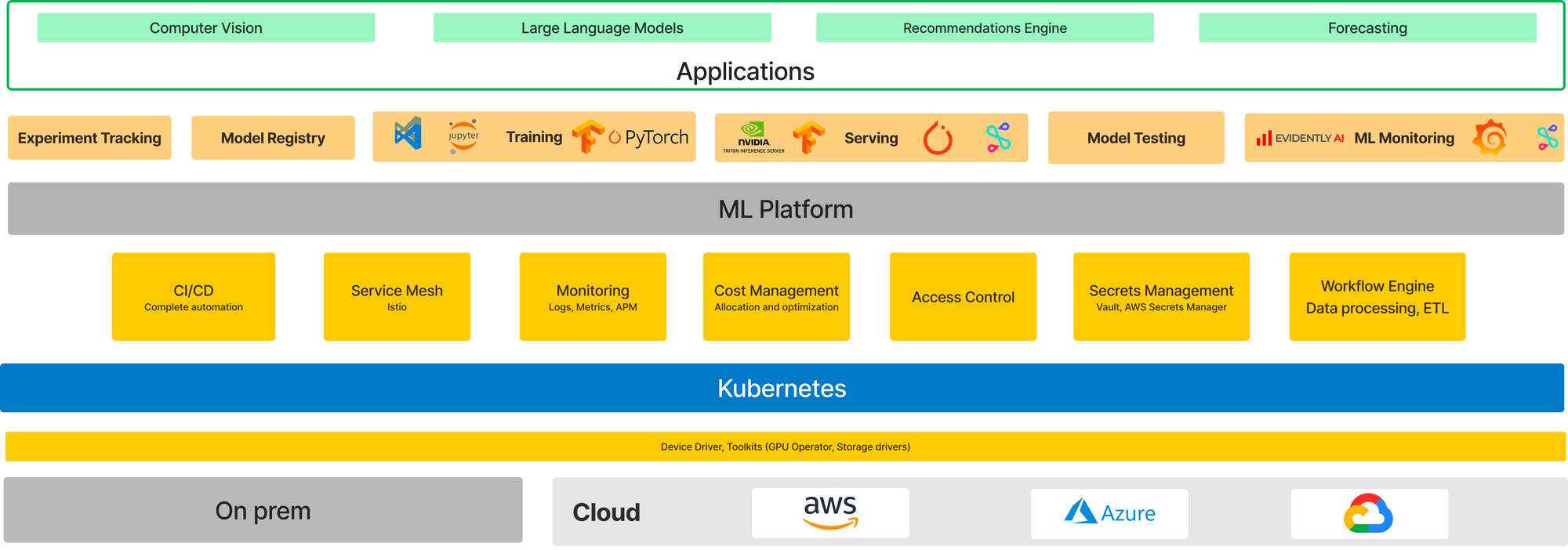
Ideally, developers should be spending more in the top green layer while the lower layers should be completely abstracted away from them. TrueFoundry provides a open and customizable stack that works with what you are currently using and helps datascientists iterate on the applications without focusing on the underlying infrastucture layers.
In the diagram below, TrueFoundryprovides the Model Training, Serving and Model registry to make it easier for datascientists to build, track and deploy models.
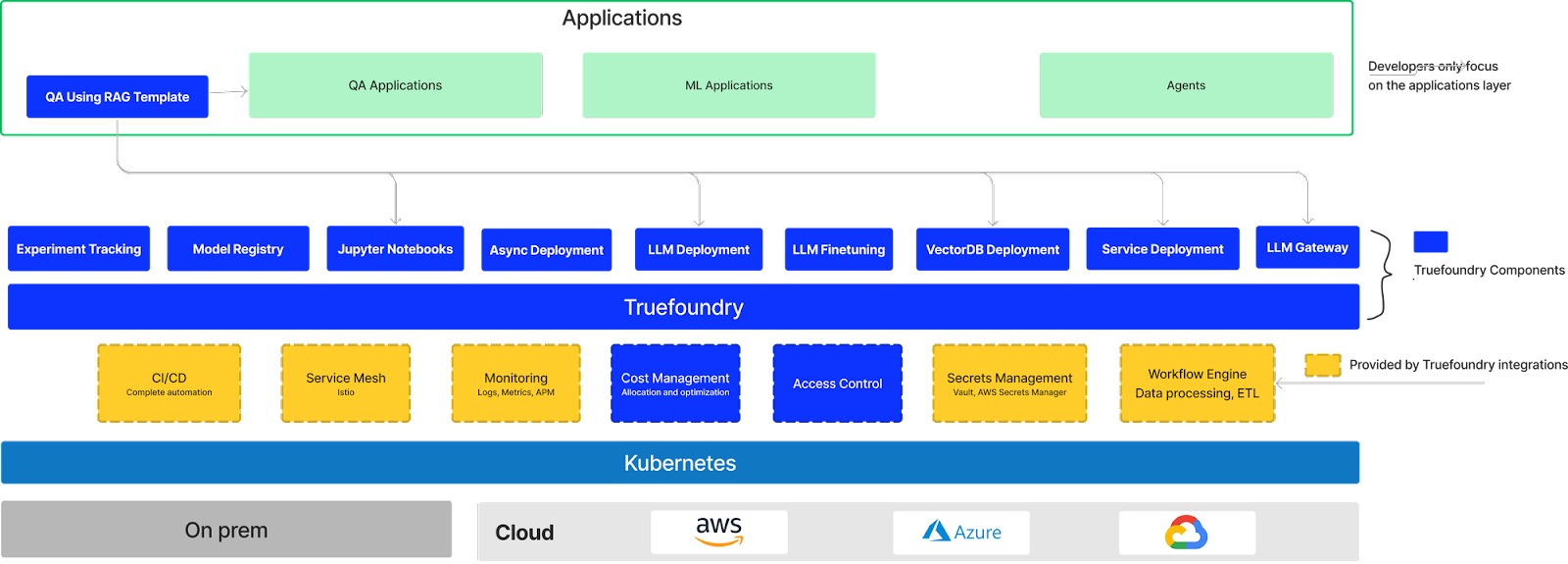
The key set of integrations that TrueFoundrycurrently provides are:
- CI/CD: Github Actions, Bitbucket Pipelines, Jenkins. We are adding additional integrations based on customer demand.
- Service Mesh: We currently operate with Istio. We do plan to support other Ingress controllers and service mesh providers like Linkerd, Nginx.
- Monitoring: The TrueFoundry deployed applications can be monitored using any of your existing monitoring systems like Prometheus, Cloudwatch, DataDog, NewRelic, ELK stack, etc.
- Cost Management: We provide fine grained cost attribution on a per service/namespace level using OpenCost. TrueFoundry also provides insights to developers directly to reduce the cost of their services.
- Access Control: TrueFoundry integrates with most IDPs like Okta, Auth0, AzureAD, Keycloak using OIDC or SAML protocols for authentication. Authorization for different workspaces is built into the product to decide permissions on a granular level.
- Secrets Management: TrueFoundry integrates with Hashcorp Vault, GCP Secrets Manager and AWS Parameter Store for secrets management. We also do plan to add the integration with Azure Vault and AWS Secrets Manager.
- Workflow Engine: TrueFoundry integrates with ArgoWorkflows to provide a workflow engine to datascientists.
TrueFoundry Architecture
TrueFoundry provides a split plane architecture which comprises of the following major components:
- Control Plane: This is the brain of the TrueFoundry system which does the orchestration of deployments across the different compute plane. We provide a hosted control plane in our usual plan. For enterprise customers, the control plane can also be deployed on the customers' cloud.
- Compute Plane: This is the Kubernetes cluster on which the user's code runs. There is an agent on the compute plane (tfy-agent) which communicates with the control plane and executes the commands received from the control plane. The user's code accessing the data runs on the compute plane - and hence the compute plane cluster should live close to data.
3. Client Interfaces: Developers and datascientists can communicate with the UI using a python SDK, or our web UI or using the TrueFoundry CLIs (servicefoundry and mlfoundry). TrueFoundry also exposes APIs for clients to build automation workflows which are documented here: https://docs.truefoundry.com/reference
4. Authentication Server: There is a central authentication and licensing server that keeps track of all the organizations and their members. This server is hosted by TrueFoundry and can also integrate with external IDPs to provide a single sign-on experience to all our users.
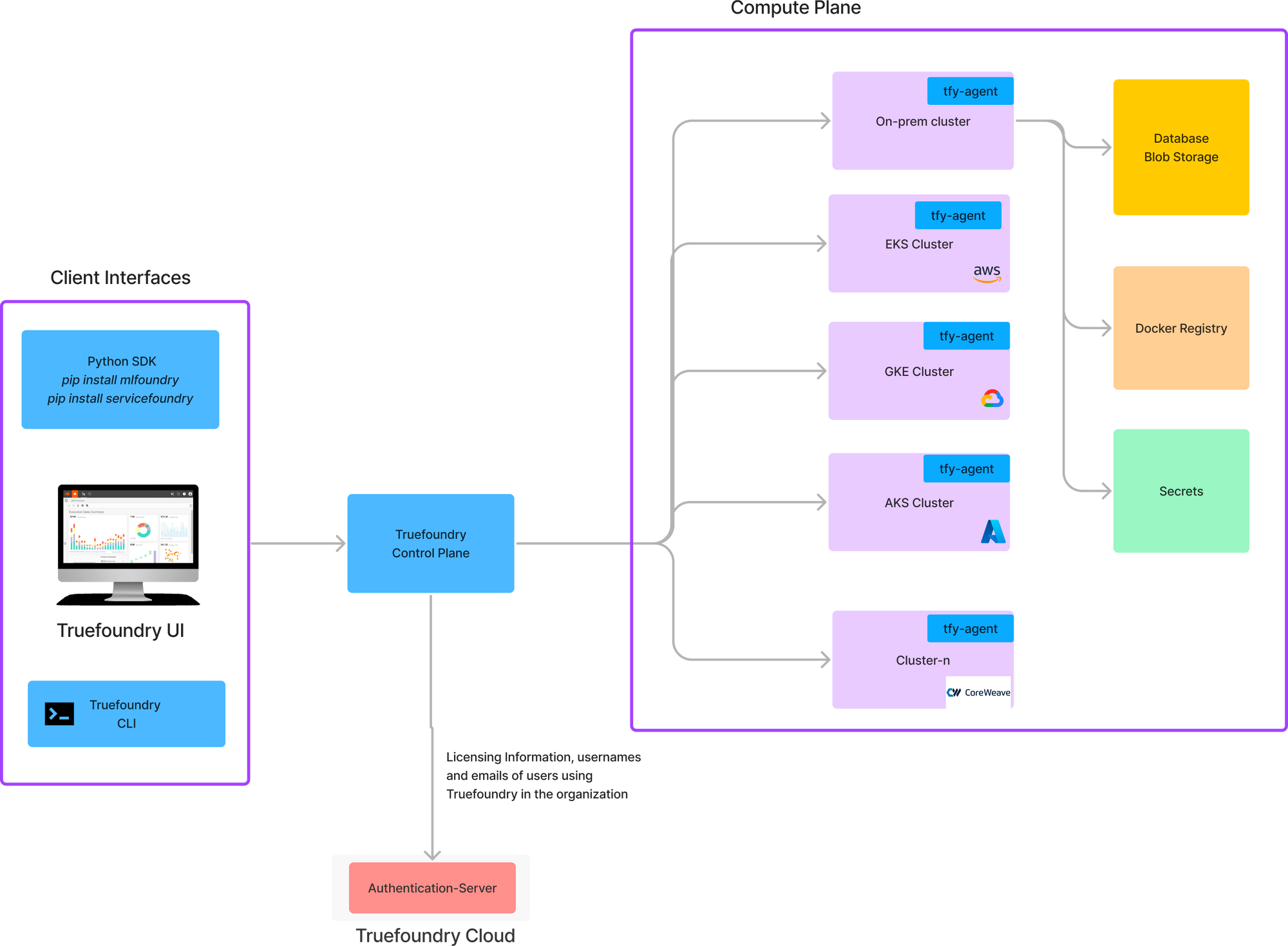
Advantages of this architecture
Secure Networking
The tfy-agent component has no ingress and is responsible for initiating the connection to the control plane. It sets up a persistent encrypted connection to the control plane over which the communication happens. This allows the system to work even if the compute-plane clusters are private or in different VPCs. The only constraint is that the control plane url should be accessible to all the compute plane clusters. You can also control the permissions granted to tfy-agent using Kubernetes RBAC to have access to certain namespaces.
Soft dependency on Truefoundry control plane
The Truefoundry control plane is only responsible for orchestrating the deployments to the compute-plane. It doesn't lie in the critical path of the request flow to the deployed services. So even if you remove the Truefoundry Control plane, all the deployed services continue to run smoothly. This decoupling of service reliability from Truefoundry helps ensure that Truefoundry doesn't lie in the critical path of service reliability.
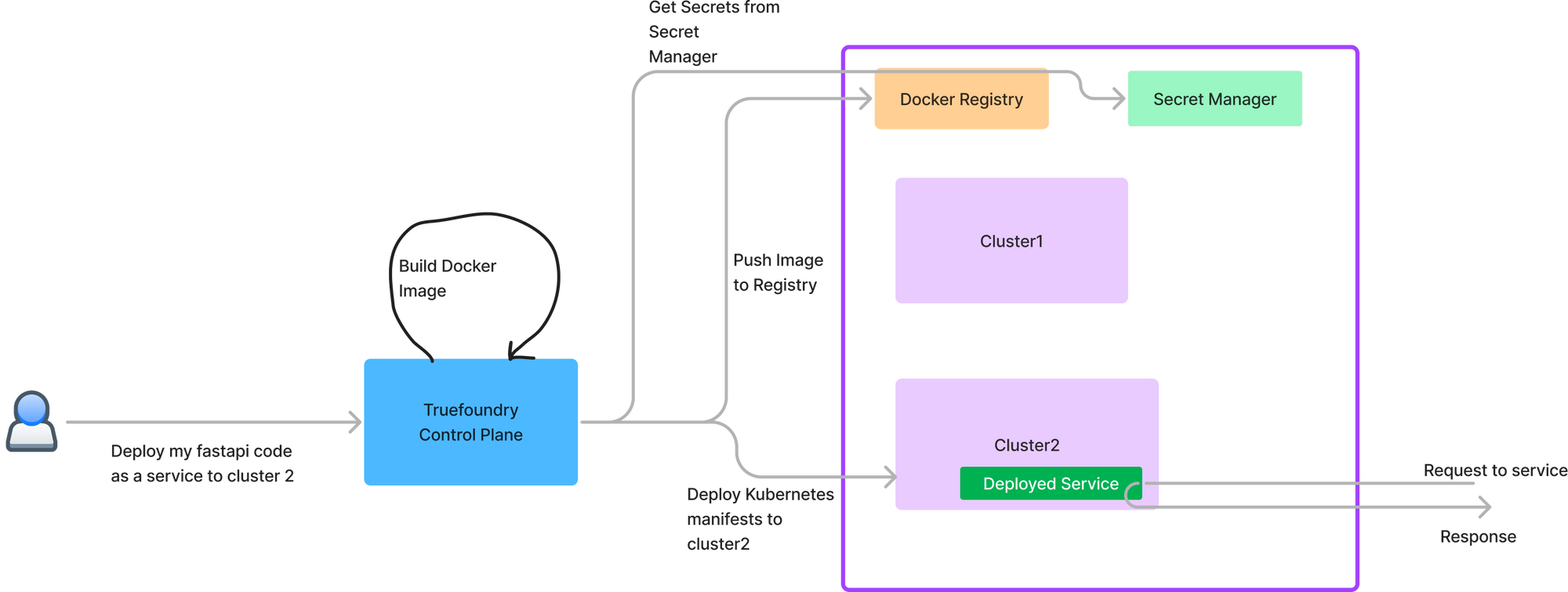
Efficient Multi-Cluster Management
Truefoundry Control Plane provides a single pane of glass to view all the kubernetes clusters across cloud providers and on-prem in the company. This also makes it quite easy to move workloads from one cluster to another using our Clone and Promote feature.
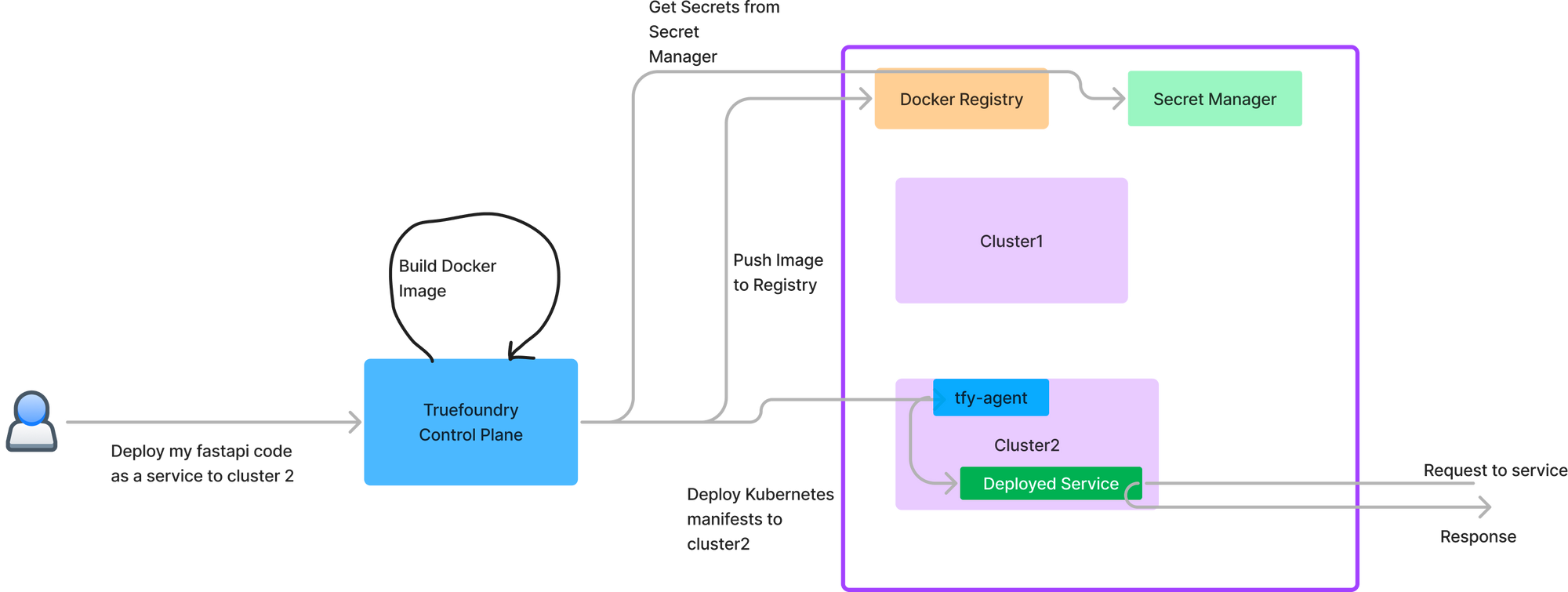
Lower cost and maintainenance
The Truefoundry agent is a very lightweight component that sits on every single cluster, while there only needs to be a single copy of the control plane. The control plane needs more resources (3CPU, 6GB RAM) while the agent needs only 0.2 CPU and 400MB RAM. As scale of traffic and teams increase, we usually need to add more clusters based on regions or teams. But the control plane doesn't need to be replicated, thus enabling lower cost and maintainenance.
A peek into the Truefoundry Control Plane
Truefoundry Control Plane comprises of multiple microservices which orchestrate the deployments, model metadata storage, etc. The key components of the Truefoundry Control plane are:
- Web UI

2. Microservices for orchestrating deployments: The control plane comprises of a few microservices to orchestrate the deployments across the clusters and also caches the live updates from all the connected clusters in compute-plane.
3. Postgres Database: This is used to store all the information about teams, services deployed and their metadata.
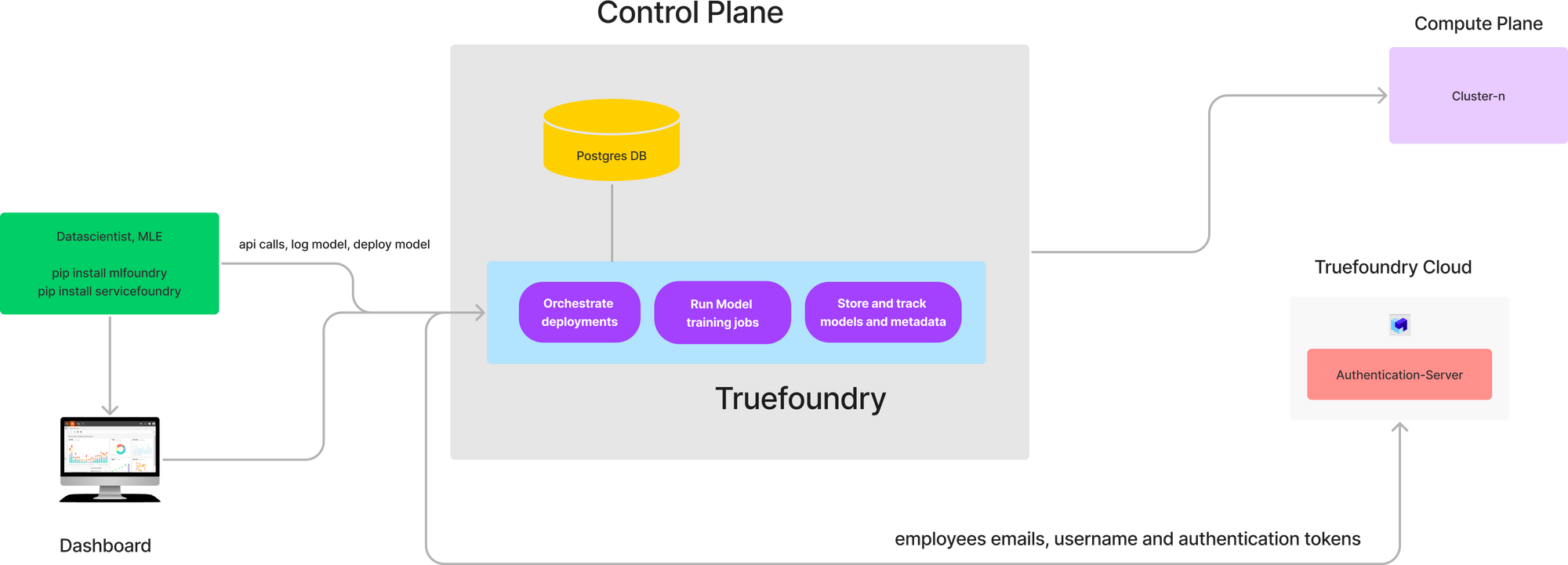
Compute-Plane Cluster
We need to install a few components on the Compute-Plane cluster to reap the full benefits of Truefoundry. The list is as follows:
- tfy-agent (Required): This is the truefoundry-agent that initiates the connection to the control-plane and helps coordinate the instructions from control-plane.
- ArgoCD (Required): ArgoCD is used to do apply all the manifests to the Kubernetes cluster. This is better than doing helm install because ArgoCD controller makes sure the internal state is synced with the desired state in the manifests and is not prone to helm installation failures.
- Istio (Required currently, will be optional in future): We currently rely on Istio as the ingress controller for the cluster. We do not mandate running istio sidecars and they can be enabled optionally if required for usecases like mutual TLS. We also plan to use the Gateway APIs of Kubernetes which will allow us to work with multiple ingress controllers like Nginx, Linkerd, Traefik, etc.
- Argo Workflows (Required only for running jobs): We use ArgoWorkflows for running all jobs inside the cluster because of the more advances options it provides when compared to Kubernetes jobs.
- Argo Rollouts (Required): We use ArgoRollouts to support Canary and BlueGreen rollouts on Kubernetes. This is currently a required prerequisite, but this will be optional in the future.
- Prometheus (Optional): This is an optional dependency needed for showing metrics like CPU, memory, request counts for the services.
- Keda (Optional): This is an optional dependency and needed if you want to enable autoscaling for your workloads.
- Loki (Optional): This helps with log aggregation and is an optional dependency. You can always use any other log aggregator that you are comfortable with like ELK Stack, Cloudwatch, Datadog, etc.
- Drivers (EFS, EBS, GPU): These are needed if you need GPU or volume support in your cluster.
- Notebook Controller (Optional): This is needed if you want to provide Notebooks on the Kubernetes cluster.
Constraints on the Kubernetes cluster AMI
Truefoundry can work with any underlying AMIs including Bottlerocket on AWS. Since the agent is just like any other helm chart running on Kubernetes, hence we don't have any constraints or requirements from the underlying AMIs and can run on any AMIs including bare metal machines.
Permissions for tfy-agent
The tfy-agent performs all actions on the Kubernetes user on behalf of the users logged into the truefoundry platform. Hence it requires admin access on a certain set of namespaces on which the users are allowed to deploy the applications. We have functionality to blacklist or whitelist a certain set of namespaces and the agent can only perform actions on those namespaces.
Authentication in Truefoundry
Truefoundry relies on a authentication server that lives on our servers for licensing and authentication.
Authorization in Truefoundry
Truefoundry provides a fine grained RBAC control at tenant, cluster, workspace and ML repo level. To understand the RBAC mechanisms in Truefoundry, you can read our docs here: https://docs.truefoundry.com/docs/collaboration-and-access-control
All the authorization rules reside in the Postgres table in the control-plane and every API call is checked to see if the user is authorized to perform that connection.
Image Build Pipeline in control-plane
Truefoundry provides a basic image building pipeline that is optimized to build images really fast on Kubernetes. In case you want to customize the pipeline of building images to include static checks or other vulnerability scanning tools, there are two approaches:
- You can continue using your own CI pipeline to build images and then provide the built image uri as the input for Truefoundry deployments.
- You can customize the Truefoundry build pipeline to include whatever components you want. Its basically a ArgoWorflow and since you own the control-plane in the Enterprise plan, you are free to customize it however you want.
Secret Management in Truefoundry
Truefoundry integrates with the most popular secret management stores. It doesn't store the secret values with it and only stores the path to those secrets.