As Generative AI becomes a core part of enterprise workflows, many organizations are reconsidering where and how their AI models run. While cloud-based services offer convenience and speed, they also raise concerns around data privacy, compliance, vendor lock-in, and long-term cost. For enterprises handling sensitive data or operating in regulated industries, on-premise GenAI offers a secure, controllable alternative. It allows businesses to run powerful language models, vector databases, and AI infrastructure within their environments. This article explores what on-premise GenAI is, why it’s gaining traction, and the platforms that make enterprise-grade deployments possible.
What is On-Premise GenAI?

On-premise generative AI refers to the deployment and execution of generative AI models, such as large language models (LLMs), image generators, or multimodal systems, within an organization’s own infrastructure. This infrastructure can include on-site data centers, private clouds, or hybrid environments, where the organization maintains full control over data flow, model access, and system security.
Unlike cloud-based GenAI solutions, which run on third-party infrastructure, on-premise deployments are designed to operate behind enterprise firewalls. This setup ensures that sensitive data never leaves the organization’s trusted environment. It also allows for fine-tuned customization of models, tighter integration with internal systems, and compliance with strict regulatory standards such as GDPR, HIPAA, or SOC 2.
On-premise GenAI solutions typically consist of several key components: pre-trained or fine-tuned LLMs, an inference engine (such as vLLM or TGI), a container orchestration platform like Kubernetes, and optional vector databases for retrieval-augmented generation (RAG) use cases.
With this setup, enterprises can deploy GenAI capabilities—such as chat assistants, summarization engines, and intelligent search without relying on external APIs or sharing data with cloud providers. This approach is particularly appealing to industries like healthcare, finance, defense, and legal services, where data privacy and infrastructure control are critical.
On-premise GenAI represents a shift from convenience to control, offering enterprises the flexibility to scale AI workloads on their terms while maintaining compliance, security, and performance standards tailored to their needs.
Why Are Enterprises Choosing On-Premise GenAI?
As Generative AI becomes more embedded in enterprise operations, many organizations are moving away from cloud-only solutions in favor of on-premise deployments. This shift is driven by the need for greater control over data, infrastructure, and long-term AI strategy.
A major reason for choosing on-premise GenAI is data privacy and compliance. Enterprises in industries such as healthcare, finance, and defense must comply with strict regulations like GDPR, HIPAA, and CCPA. Cloud-based services often raise concerns about where data is stored, how it is accessed, and who has visibility into sensitive information. On-premise deployment keeps data within the organization's environment, improving auditability and reducing exposure.
Another significant factor is customization and control. Enterprises frequently need to fine-tune models, enforce strict output behavior, or integrate with internal systems. On-premise GenAI allows organizations to modify model pipelines, manage prompt behavior, and deploy domain-specific enhancements without relying on third-party APIs or external release cycles.
Avoiding vendor lock-in is also a strong motivator. Relying exclusively on a single cloud provider limits flexibility and can introduce long-term cost and innovation constraints. On-premise setups offer full-stack ownership, allowing teams to swap components, test open-source models, and evolve their architecture without external dependencies.
Cost predictability and optimization are equally important. For businesses running large-scale inference workloads, usage-based billing can become difficult to manage. With on-premise infrastructure, costs are tied to hardware usage rather than per-token or per-request fees, making financial planning more transparent.
On-premise GenAI offers enterprises the ability to operate AI workloads securely, flexibly, and cost-effectively while maintaining compliance and avoiding reliance on external service providers.
Core Infrastructure Required for On-Premise GenAI
Running Generative AI on-premise requires a well-architected stack that balances performance, control, and scalability. Below are the key infrastructure components required to deploy GenAI systems within enterprise environments.
- High-Performance Hardware:
Powerful GPUs are essential for efficient LLM inference. Organizations typically use NVIDIA A100, H100, or L40 GPUs depending on the workload. These GPUs offer the memory bandwidth and compute power needed to handle large models and concurrent requests. AI accelerators like Intel Habana or AMD Instinct can also be considered based on compatibility and budget. - Inference Engine:
An optimized inference engine like vLLM, TGI, or DeepSpeed-Inference is needed to serve models with low latency. These engines support features like batch processing, token streaming, and KV caching, making them ideal for high-throughput, real-time applications. - Container Orchestration:
Kubernetes and Docker are widely used to deploy and manage inference services. They provide scalability, fault tolerance, and resource management across multiple nodes. This orchestration layer ensures that inference workloads remain stable and responsive under varying traffic loads. - Vector Database (Optional):
For use cases like retrieval-augmented generation (RAG), vector databases such as FAISS, Qdrant, or Weaviate are used to store and search embeddings. These systems allow GenAI models to ground their outputs in enterprise-specific knowledge bases. - Monitoring and Observability:
Tools like Prometheus, Grafana, and OpenTelemetry help monitor GPU usage, request latency, error rates, and throughput. Observability is crucial to maintaining performance, detecting bottlenecks, and optimizing resource allocation over time.
Together, these components form the foundation of a robust, scalable, on-premise GenAI deployment.
Challenges of On-Premise AI Deployment
While on-premise GenAI offers greater control and data security, it comes with its own set of challenges that enterprises must plan for carefully. These challenges span infrastructure, scalability, operations, and maintenance.
Hardware Procurement and Setup: Acquiring and maintaining GPUs or specialized AI accelerators can be time-consuming and costly. Lead times for high-end hardware like NVIDIA A100s or H100s can extend for months, and setting up cooling, power, and rack space adds additional complexity.
Infrastructure and DevOps Complexity: Running large models on-premise requires managing container orchestration, GPU scheduling, networking, and resource limits. Without a dedicated DevOps or MLOps team, ensuring uptime and scalability can become a bottleneck, especially as usage grows.
Scaling and Load Management: Autoscaling is more complex in on-premise environments compared to cloud platforms. Enterprises must plan for peak load scenarios and build in buffer capacity, which can lead to underutilization and increased costs if not optimized properly.
Model Management and Versioning: Hosting multiple models for different teams or use cases requires version control, rollback support, and secure access control. Without proper tooling, model sprawl can lead to instability and inefficiency in deployment pipelines.
Monitoring and Troubleshooting: Identifying bottlenecks, latency spikes, or memory issues requires real-time monitoring tools. Without robust observability in place, diagnosing performance problems becomes reactive rather than proactive.
Despite these challenges, many enterprises successfully deploy GenAI on-premise by investing in the right tools, platforms, and operational practices from the start.
Top 5 Platforms for On-Premise Gen AI
Choosing the right platform is essential to successfully deploy and manage GenAI workloads on-premise. These platforms help abstract the complexities of infrastructure, orchestration, and model serving. Below are some of the top solutions built for secure, scalable, and customizable on-premise GenAI deployments.
1. TrueFoundry
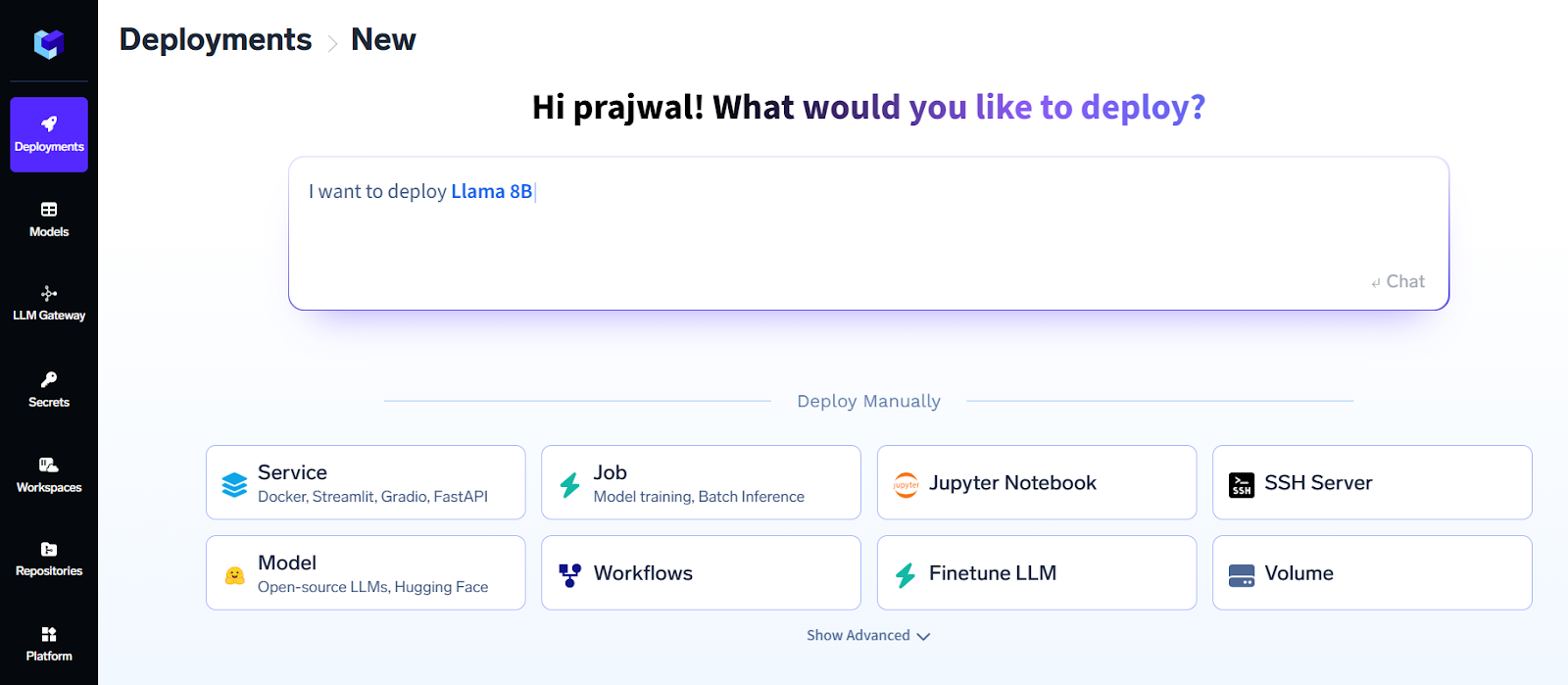
TrueFoundry is an enterprise-grade, Kubernetes-native platform designed to streamline the deployment, inference, and scaling of AI and GenAI workloads across cloud and on-premise environments. It abstracts away the complexity of managing LLM infrastructure by offering a robust AI Gateway, optimized model serving layers, and full-stack MLOps integration.
Built with a developer-first mindset, TrueFoundry empowers ML and platform teams to focus on building and optimizing models rather than managing compute infrastructure. It offers seamless integrations with leading inference frameworks like vLLM and Text Generation Inference (TGI), supporting lightning-fast, token-efficient LLM deployments.
Top Features:
Unified AI Gateway: Serve 250+ open-source and proprietary LLMs using a consistent OpenAI-compatible API layer. Switch between models like LLaMA 2, Mistral, Mixtral, Claude, and GPT via multi-model routing—all without changing your integration code.
Kubernetes-Native Architecture: Automatically scales LLM inference workloads across any environment—AWS, GCP, Azure, or on-prem using native Kubernetes orchestration. Comes prebuilt with Helm-based GitOps support for declarative infrastructure management.
Optimized for Inference at Scale: TrueFoundry natively integrates with vLLM to deliver sub-400ms latency and serve 100+ concurrent users per GPU. Mixed precision, quantization, and batching are supported out of the box to lower the cost per token.
Full Observability & Control: Real-time token-level analytics, latency metrics, rate limiting, and automatic load balancing give engineering teams full insight and control over production inference.
Prompt & Version Management: Manage, version, and test prompts directly within the platform. Enable A/B testing and rollback support for rapid iteration and experimentation.
Security & Compliance: Deploy LLMs in your VPC or on-prem with built-in RBAC, encrypted communication, and SOC2-compliant practices. No model or prompt data ever leaves your infrastructure.
2. NVIDIA Enterprise AI
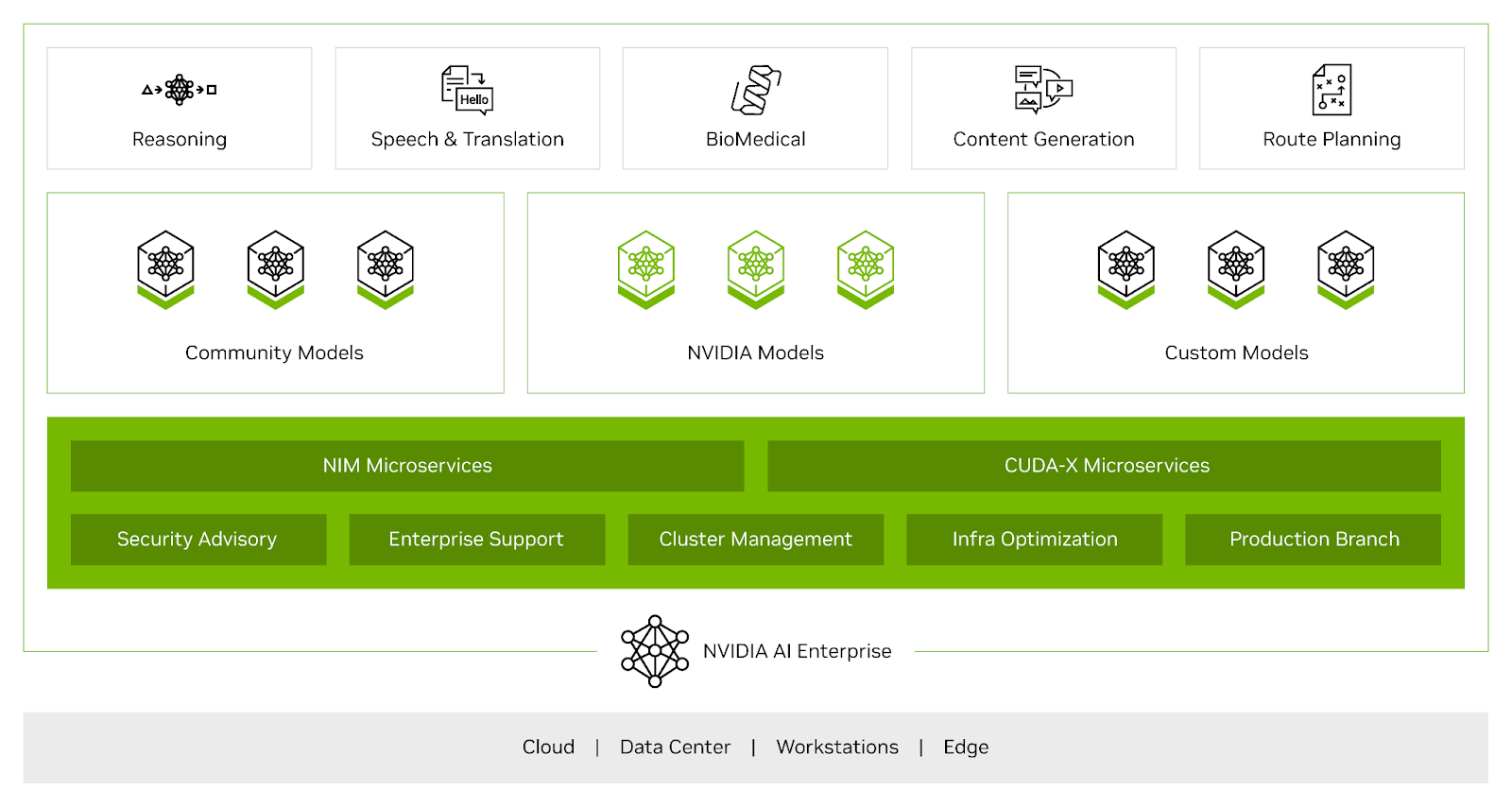
NVIDIA Enterprise AI is a full-stack suite of software and GPU-accelerated infrastructure built to power scalable AI, machine learning, and generative AI workloads in enterprise environments. Designed for hybrid and on-premise deployment, it enables organizations to run state-of-the-art AI, including LLMs, computer vision, and predictive analytics, on NVIDIA-certified systems using NVIDIA AI Enterprise, a software layer optimized for performance, security, and support.
Whether deploying models in private data centers or on hybrid cloud infrastructure, NVIDIA Enterprise AI empowers enterprises to build secure, low-latency AI applications without sending data outside their network. It is ideal for industries like healthcare, finance, and manufacturing that require on-premise AI with full data sovereignty.
Top Features
- Full-Stack AI Software Suite: Includes Triton Inference Server, TensorRT, and NeMo for high-performance model training and deployment.
- Hybrid & On-Premise Flexibility: Deploy across VMware, Red Hat OpenShift, or bare metal in secure, data-sovereign environments.
- GPU-Optimized Performance: Designed to leverage NVIDIA enterprise GPUs (A100, H100, L40) for low-latency, high-throughput inference.
3. Red Hat OpenShift AI
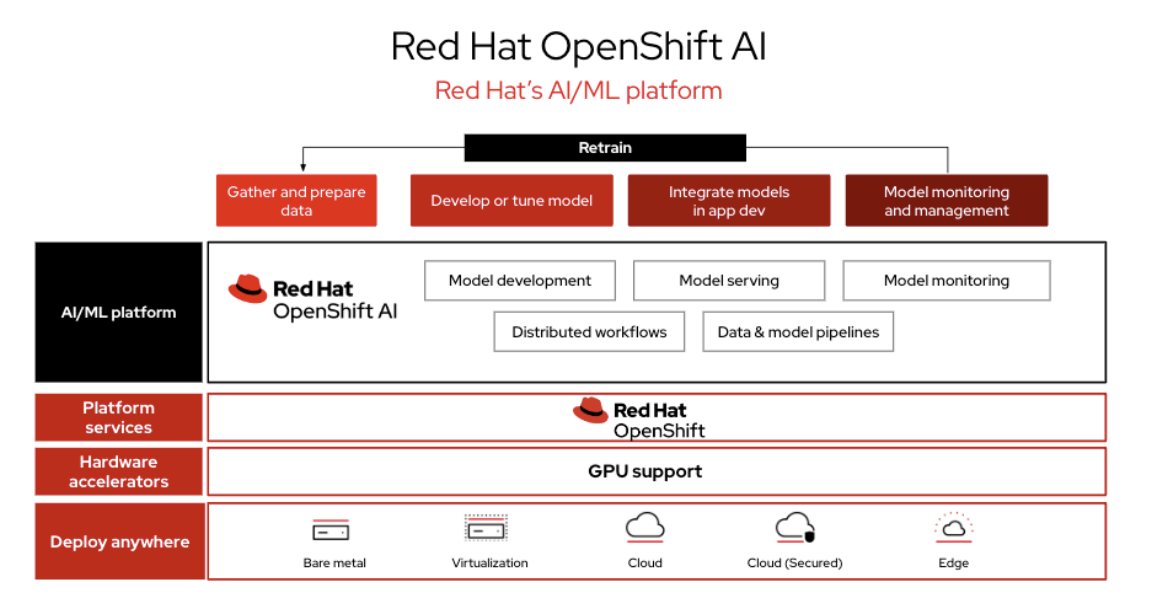
Red Hat OpenShift AI (formerly Red Hat OpenShift Data Science) is an enterprise-ready MLOps platform that enables organizations to build, train, deploy, and monitor AI/ML models across hybrid cloud environments. Built on Kubernetes via OpenShift, it offers a fully integrated environment for model development and production, with flexibility for on-premise, cloud, or edge deployments.
OpenShift AI brings together open-source tools and enterprise support, enabling seamless collaboration between data scientists, ML engineers, and DevOps teams. It supports custom models as well as integration with open-source and commercial LLMs, offering secure and compliant infrastructure for sensitive workloads.
Top Features
- Hybrid MLOps Platform: Run AI/ML workloads consistently across on-prem, cloud, or edge using Kubernetes-native orchestration.
- Built-in Tooling & Notebooks: Includes JupyterHub, model registry, pipelines, and CI/CD integration for end-to-end workflows.
- Enterprise-Grade Security: Offers RBAC, audit logging, and compliance-ready infrastructure with Red Hat support.
4. Ray (Anyscale)
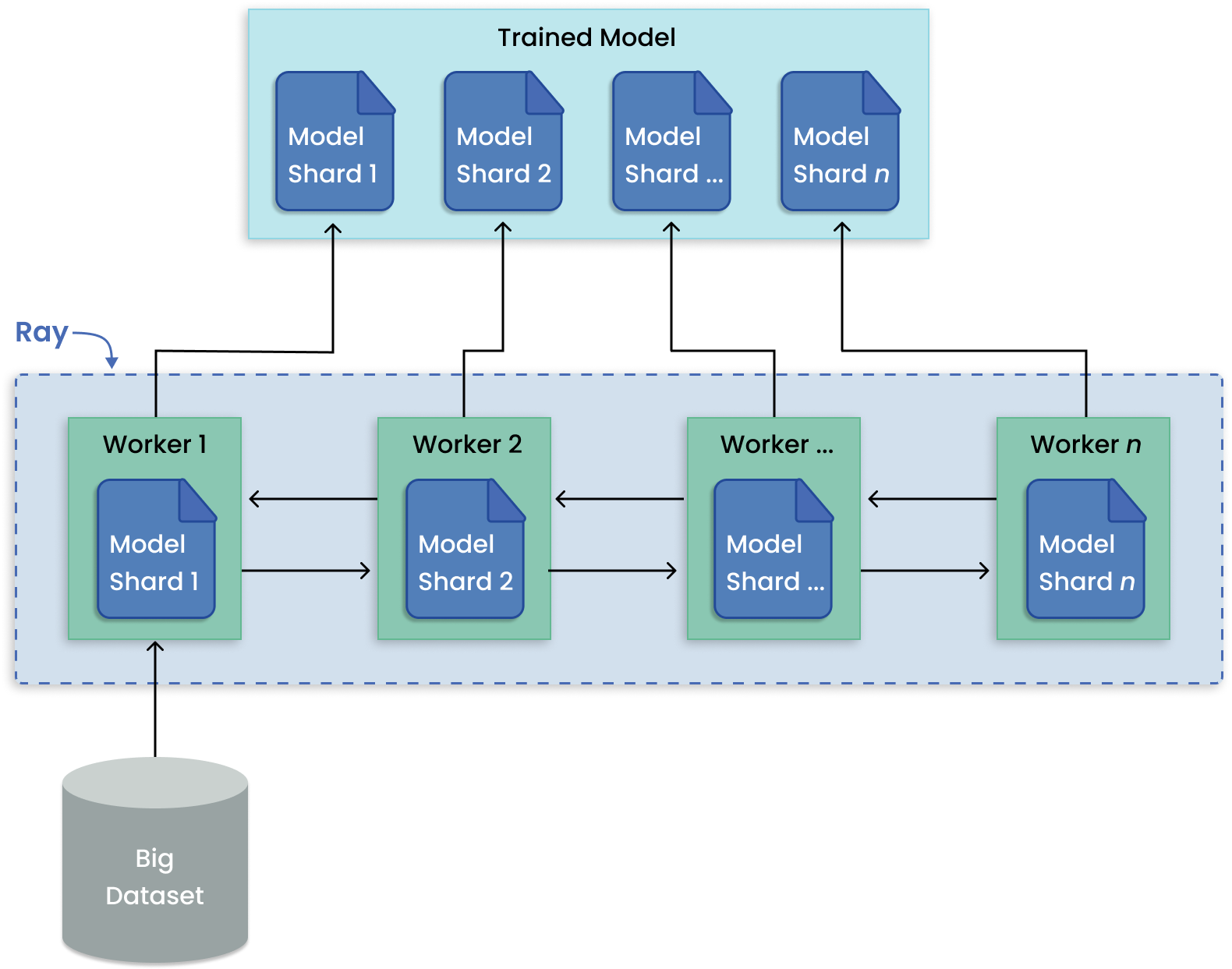
Ray is a scalable, distributed computing framework designed for building and deploying AI applications—from training LLMs to serving them with high throughput. It can be deployed entirely on-premise, making it a great choice for organizations needing full control over infrastructure.
Ray powers modern AI stacks like vLLM, DeepSpeed, and Hugging Face Transformers, and supports scalable inference via Ray Serve. With native support for Kubernetes and GPU scheduling, Ray clusters can run securely in private data centers for LLMOps workloads.
Top Features:
- On-Prem Distributed AI Orchestration: Deploy Ray on your own clusters to manage training, tuning, and inference pipelines at scale.
- LLM-Ready Stack: Compatible with vLLM, Hugging Face, DeepSpeed, and LangChain-based applications.
- Ray Serve for Inference: Serve models with dynamic autoscaling, routing, and high concurrency on GPU nodes.
5. IBM Watson.ai

IBM Watson AI is a suite of AI services and tooling designed to help enterprises integrate artificial intelligence into their workflows with a strong emphasis on trust, transparency, and governance. Now part of IBM watsonx, the platform includes watsonx.ai for building and tuning models, watsonx.data for scalable data management, and watsonx.governance to ensure responsible AI usage across the lifecycle.
Watson AI is optimized for hybrid cloud and on-premise environments using IBM Cloud Pak for Data and Red Hat OpenShift, giving enterprises full control over their data and model deployment. It supports foundation models, custom model fine-tuning, and integration with open-source LLMs, all backed by enterprise SLAs.
Top Features
- watsonx Platform: Unified stack with watsonx.ai (model building), watsonx.data (governed data store), and watsonx.governance (AI governance).
- Hybrid & On-Prem Deployment: Deploy on IBM Cloud, AWS, or your own infrastructure using OpenShift and Cloud Pak for Data.
- Trust-Centric AI: Built-in tools for explainability, bias detection, model lineage, and regulatory compliance.
On-Premise Gen AI vs Cloud Gen AI: The Difference
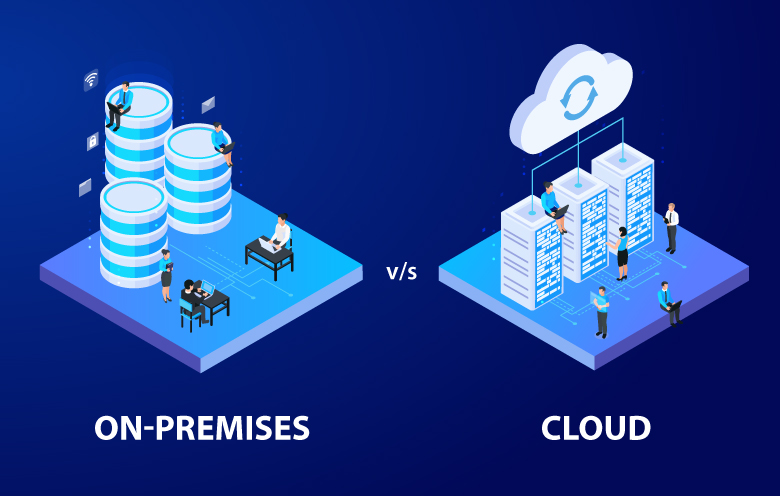
On-Premise GenAI offers enterprises full control over their infrastructure, models, and data. It is the preferred choice for organizations operating in highly regulated industries such as healthcare, finance, or government, where compliance with privacy standards like GDPR and HIPAA is critical. On-premise deployments allow teams to keep sensitive data within their own environments, customize model behavior, and optimize infrastructure for long-term cost predictability. While the initial setup may require more effort and investment, it provides greater flexibility, auditability, and integration with internal systems.
Cloud GenAI, on the other hand, offers speed, scalability, and convenience. It allows teams to quickly prototype and deploy AI applications without worrying about infrastructure management. Cloud providers handle autoscaling, hardware provisioning, and model updates, enabling faster time to market. However, it may come with concerns around data residency, vendor lock-in, and unpredictable usage-based pricing. For many organizations, the choice comes down to balancing compliance needs with operational agility.
Conclusion
In conclusion, on-premise Generative AI is no longer just a fallback for regulated industries. It is quickly becoming a strategic advantage for enterprises that require data sovereignty, infrastructure control, and long-term scalability. Solutions like TrueFoundry, NVIDIA Enterprise AI, Red Hat OpenShift AI, IBM Watson AI, and Ray offer powerful, production-grade capabilities for building and serving GenAI models entirely within a company’s own infrastructure.
As AI systems grow more complex and data sensitivity increases, on-premise deployment provides a future-ready foundation. It enables organizations to innovate with confidence, stay compliant, and maintain full ownership of both their models and data.