Large Language Models (LLMs) are transforming how enterprises automate tasks, generate content, and interact with data. However, most LLM services today are cloud-centric, raising concerns about data security, compliance, and control. For organizations dealing with sensitive or regulated information, relying on external APIs or public cloud models is often not viable. This has led to a growing shift toward on-premise LLM deployments, where businesses run models securely within their own infrastructure. In this article, we explore what on-premise LLMs are, why they matter, how they work, and how platforms like TrueFoundry enable scalable, secure deployments in enterprise environments.
What are On-Premise LLMs?
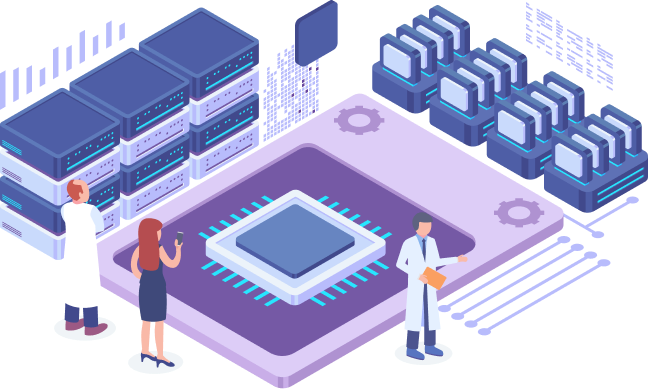
On-premise LLMs refer to large language models that are deployed and operated within an organization’s own infrastructure rather than through external cloud services or third-party APIs. These models can be open-source or proprietary and are typically run on in-house GPU servers, private data centers, or isolated cloud environments configured to meet internal security and compliance standards.
Unlike cloud-hosted LLMs that rely on public endpoints and vendor-managed infrastructure, on-premise LLMs are fully controlled by the organization. This allows for greater customization, fine-tuning, and integration with internal systems and workflows. Enterprises can choose which models to use, such as LLaMA 2, Mistral, or Mixtral, and optimize them based on specific business or domain needs.
On-premise deployment enables teams to tailor model behavior, enforce data residency policies, and ensure that sensitive information never leaves their trusted network. It also opens up opportunities for tighter performance tuning and cost control, especially for high-volume or latency-sensitive applications. For organizations prioritizing autonomy, security, and regulatory compliance, on-premise LLMs offer a practical and scalable alternative to commercial AI APIs.
Limitations of Cloud-Centric AI
Cloud-based AI services have made it easy for teams to experiment, prototype, and deploy machine learning models at scale. However, when it comes to production workloads in enterprise environments, relying solely on cloud-centric LLMs presents several limitations that can’t be ignored.
Data privacy and control are the most significant concerns. When using public cloud APIs, sensitive input data must be transmitted over the internet and processed on external infrastructure. This introduces risks around data leakage, unauthorized access, and compliance violations—especially in sectors like healthcare, finance, defense, and legal services, where strict regulatory standards apply.
Vendor lock-in is another major drawback. Cloud AI platforms often bundle inference APIs, storage, and fine-tuning into proprietary ecosystems. Once a workflow is built around a specific provider, migrating to another service or bringing workloads in-house becomes time-consuming and costly. This dependency limits long-term flexibility and control over model updates or usage terms.
Unpredictable cost scaling also becomes a challenge as usage grows. LLMs are compute-intensive, and cloud pricing models based on token counts or request volume can lead to spiraling operational costs—especially for applications with high throughput or constant interaction.
Additionally, cloud environments offer limited options for low-latency and edge deployments. Applications that require near-instant responses or offline functionality may struggle to meet performance targets when dependent on external APIs.
Lastly, cloud providers abstract away much of the infrastructure, leaving teams with minimal visibility into performance bottlenecks, optimization opportunities, or tuning parameters.
For enterprises that demand control, transparency, and long-term sustainability, these limitations make a strong case for adopting on-premise LLM solutions.
Ensure Data Privacy with On-Premise LLMs
- Local data processing: On-premise LLMs process all data within your private infrastructure. Nothing leaves your environment, which means sensitive inputs like financial records, medical data, or intellectual property remain completely secure.
- Regulatory compliance: Hosting LLMs internally helps meet data protection requirements under GDPR, HIPAA, and CCPA. Enterprises can enforce strict access controls, log all activity, and maintain audit trails to prove compliance.
- No third-party exposure: Unlike public cloud APIs, on-premise systems eliminate the need to send data to external providers. This reduces the attack surface and mitigates the risk of data leaks or unauthorized access.
- Policy-driven deployment: In industries with rigid data classification rules, on-premise LLMs offer a safe way to integrate AI without violating internal or external policies on data sharing or storage.
- Custom privacy enhancements: Enterprises can implement advanced safeguards such as encryption, private fine-tuning, and content filtering tailored to their risk tolerance and security posture.
- Unlocking innovation securely: With full control over data flow and inference logic, teams can confidently explore advanced GenAI applications without privacy concerns getting in the way.
Technical Architecture of On-Premise LLMs
Deploying LLMs on-premise requires a carefully structured architecture that balances performance, security, and maintainability. Below are the key components typically found in a production-grade setup.
Compute Infrastructure: High-performance GPUs are the foundation of on-premise LLMs. Enterprises often use NVIDIA A100, H100, or L40 GPUs, depending on the model size and throughput requirements. These are hosted in local data centers or private cloud clusters with appropriate cooling, networking, and storage.
Inference Engine: Inference frameworks such as vLLM, TGI, or DeepSpeed-Inference handle the actual model execution. They optimize memory use, support token streaming, and allow for batching multiple requests to maximize throughput.
Model Management and Storage: Models are stored locally in secure artifact repositories or volume mounts. Versioning, rollback, and access control mechanisms are essential to manage model lifecycles and audit changes.
Containerization and Orchestration: Tools like Docker and Kubernetes are used to deploy, scale, and manage LLM workloads. Kubernetes handles autoscaling, GPU scheduling, load balancing, and failure recovery, ensuring consistent performance across services.
API Layer and Routing: A REST or OpenAI-compatible API layer exposes LLM functionality to internal applications. It can include multi-model routing, user authentication, and prompt filtering for safety and control.
Observability and Monitoring: Metrics such as latency, GPU utilization, request throughput, and token generation speed are tracked using tools like Prometheus, Grafana, and OpenTelemetry. Logging and alerting are critical for maintaining uptime and debugging issues.
This modular architecture allows enterprises to build scalable and secure LLM systems tailored to their internal policies, performance targets, and compliance requirements.
Real-World Use Cases for On-Premise LLMs
On-premise LLMs are gaining adoption across industries that require data sovereignty, low-latency performance, and full control over AI pipelines. Here are some common and impactful use cases.
Healthcare
Hospitals and research labs use LLMs to summarize patient notes, generate discharge reports, and assist with clinical documentation. On-premise deployments ensure patient health information stays within the hospital’s secure infrastructure, supporting HIPAA compliance and institutional data policies.
Banking and Finance
Financial institutions use LLMs for tasks such as summarizing earnings calls, automating compliance reports, and analyzing financial statements. On-premise setups prevent sensitive financial data from being exposed to third-party APIs while maintaining compliance with internal risk frameworks and regulatory audits.
Government and Defense
Agencies use LLMs for question answering, summarization of classified reports, and internal knowledge retrieval. Since national security data must remain strictly contained, on-premise LLMs enable generative AI applications without violating data classification protocols.
Legal
Law firms and legal departments use LLMs to analyze contracts, generate summaries, and assist with legal research. On-premise deployment ensures attorney–client privileged information never leaves internal servers, maintaining confidentiality and meeting bar association requirements.
Manufacturing
Enterprises in manufacturing use LLMs to generate troubleshooting guides, interpret sensor logs, and assist field technicians. Deploying LLMs on local servers helps avoid sending proprietary machine data to external services and reduces latency for remote or disconnected environments.
Telecommunications
Telecom companies use LLMs to power chatbots, triage tickets, and provide automated service recommendations. On-premise deployment enables real-time performance while keeping customer data within the internal infrastructure for compliance with regional privacy laws.
These use cases highlight how on-premise LLMs unlock powerful automation and intelligence without compromising on security, compliance, or control.
On-Premise LLM Deployment Workflow
Deploying LLMs on-premise involves a series of coordinated steps, from model selection to production monitoring. A well-defined workflow ensures the system is scalable, secure, and optimized for your organization’s needs.
1. Model Selection and Preparation
The process begins by choosing an appropriate model based on your use case. Popular open-source models like LLaMA 2, Mistral, or Mixtral are often preferred for on-premise deployments. Once selected, the model is downloaded, quantized if needed, and validated for compatibility with your infrastructure.
2. Infrastructure Provisioning
Next, GPU servers or private cloud resources are prepared. This includes setting up container runtimes (e.g., Docker), orchestrators (e.g., Kubernetes), and storage layers to host model weights and logs. Access controls and security policies are configured to meet compliance requirements.
3. Inference Engine Integration
The model is loaded into an inference engine such as vLLM or TGI. These engines provide the runtime environment for real-time text generation, batching, streaming, and memory optimization. Configuration files define batch size, max tokens, and concurrency limits.
4. API Exposure and Routing
Once the engine is operational, it is exposed through a REST or OpenAI-compatible API. This allows internal apps, tools, or user interfaces to query the model. Multi-model routing, authentication, and rate limiting may be added at this layer for better control.
5. Monitoring and Scaling
Observability tools are connected to track GPU usage, latency, token throughput, and request errors. Based on traffic patterns, autoscaling policies or manual scaling procedures are configured to handle demand without downtime.
Following this workflow helps organizations launch and manage LLMs efficiently within their infrastructure without relying on external platforms or exposing sensitive data.
Advantages & Challenges of On-Premise LLMs
Deploying LLMs on-premise gives organizations full control over their AI infrastructure but also comes with trade-offs. Understanding both the benefits and challenges is essential for successful adoption.
Advantages
- Data Control and Privacy
Organizations retain complete ownership of all input and output data. This eliminates risks associated with sending sensitive information to external APIs and helps ensure compliance with data protection regulations. - Customization and Fine-Tuning
On-premise environments allow full access to model weights and architecture. Teams can fine-tune models on proprietary datasets, modify tokenization logic, and build highly tailored AI experiences. - Cost Predictability
While initial setup costs may be higher, ongoing usage is not tied to per-token or per-request billing. This makes cost planning more predictable for high-volume or always-on applications. - Performance Optimization
Enterprises can optimize for latency, throughput, or memory usage based on their own infrastructure and use cases, enabling better performance for specific workloads.
Challenges
- Infrastructure Complexity
Setting up and maintaining GPU clusters, inference engines, and orchestration tools requires DevOps expertise and continuous oversight. - Scaling and Load Management
Unlike cloud platforms that auto-scale on demand, on-premise systems require advance capacity planning and often manual scaling strategies. - Model Updates and Maintenance
Staying up to date with the latest model improvements or security patches requires a structured MLOps process. This responsibility lies entirely with the internal team. - Initial Capital Investment
Procuring and configuring GPU servers, storage, and networking equipment involves upfront costs that may be significant for some organizations.
How TrueFoundry Assists in On-Premise LLM Deployments
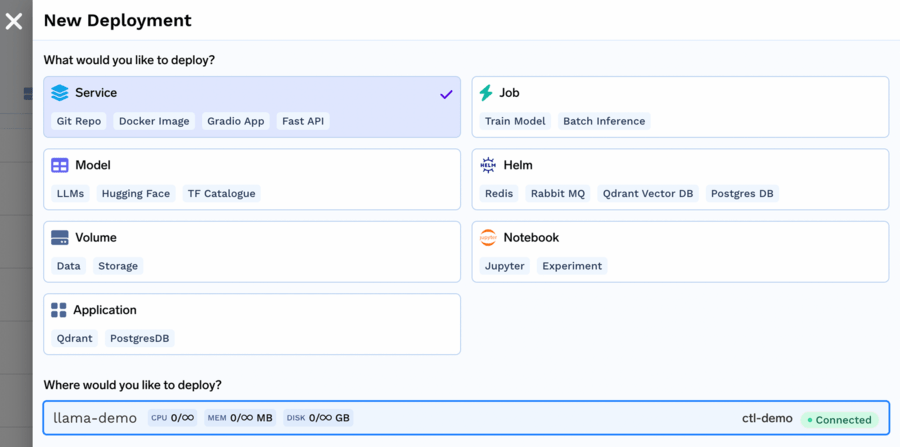
TrueFoundry simplifies the deployment and management of large language models within private infrastructure, making on-premise GenAI accessible to enterprises without requiring deep DevOps or MLOps expertise. Built on top of Kubernetes, TrueFoundry enables fast, secure, and scalable LLM serving through pre-integrated support for high-performance inference engines like vLLM and TGI.
The platform abstracts the complexity of managing containers, GPUs, and scaling policies, allowing teams to focus on building applications rather than maintaining infrastructure. With TrueFoundry’s AI Gateway, organizations can expose LLMs using OpenAI-compatible APIs while applying rate limiting, token-based billing, and multi-model routing, all within their secure environment.
TrueFoundry also offers built-in observability, including real-time monitoring of token usage, latency, and model performance. This helps teams optimize throughput, troubleshoot issues, and enforce governance.
Whether deploying LLaMA 2, Mistral, or fine-tuned internal models, TrueFoundry gives enterprises a production-ready solution for on-premise GenAI, fully customizable, compliant, and built to scale.
- Prebuilt LLM deployment workflows for vLLM and TGI
- OpenAI-compatible API gateway with enterprise controls
- Integrated monitoring, prompt tracking, and resource visibility
Conclusion
As enterprises increasingly adopt large language models, on-premise deployment offers a secure and flexible path to harness GenAI without compromising data privacy, compliance, or infrastructure control. While cloud-based solutions provide convenience, they often fall short in regulated or sensitive environments. On-premise LLMs give organizations full ownership of the stack, greater customization, and predictable costs, making them ideal for long-term AI strategies. With platforms like TrueFoundry, deploying and scaling LLMs internally becomes faster, more efficient, and easier to manage. For organizations focused on control, transparency, and innovation, on-premise GenAI is not just an alternative, it is a strategic advantage.