Large Language Models (LLMs) have transformed how we build applications, from chatbots and AI copilots to complex enterprise systems. While model training often gets the spotlight, inference drives performance, cost, and user experience in production. Inference refers to the real-time generation of outputs when a model is used, not trained. As the adoption of LLMs grows, teams face increasing challenges related to latency, GPU limitations, and scaling costs. Optimizing LLM inference has become essential. In this article, we explore what LLM inference is, key optimization techniques, infrastructure challenges, and how TrueFoundry helps scale inference efficiently.
What is LLM Inference?
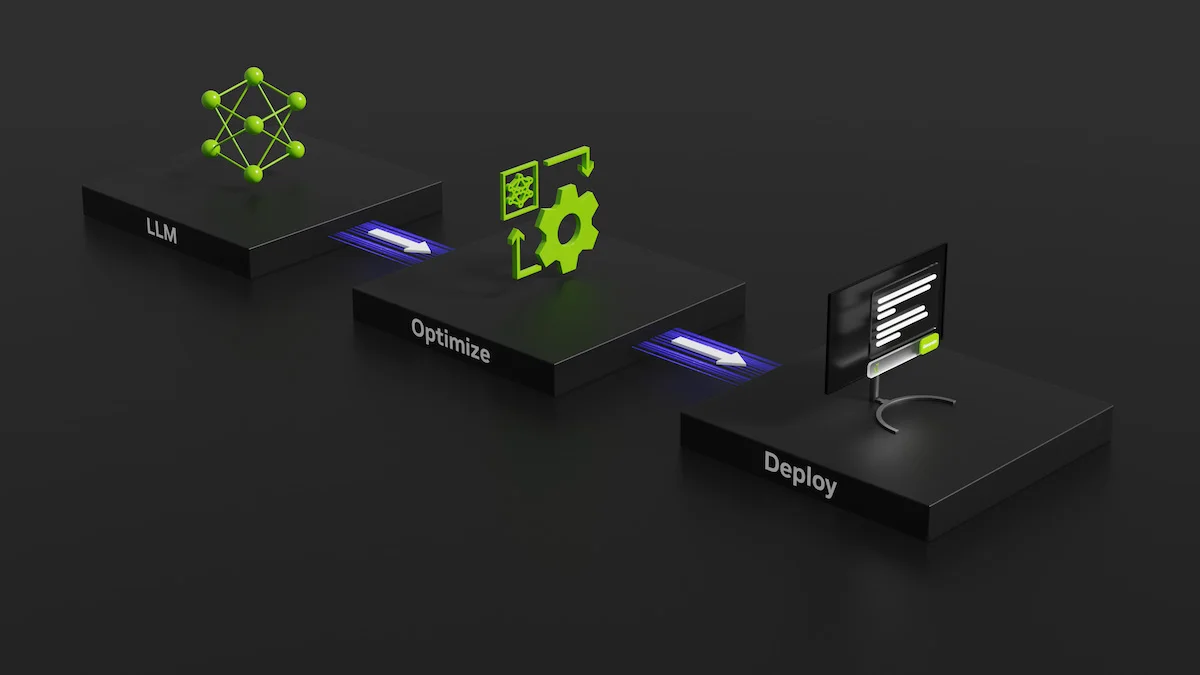
LLM inference is the process of using a pre-trained large language model to generate outputs based on user input. Unlike training, which updates model weights, inference is a forward-pass operation that computes the next token or sequence of tokens based on the input prompt. This process happens every time a user interacts with an AI application powered by an LLM.
At its core, inference begins with tokenization, where the input text is broken down into tokens that the model understands. These tokens are then passed through the model’s transformer layers, which apply learned weights to produce contextual embeddings. Finally, a decoding strategy (like greedy search or beam search) generates the next most likely token, continuing until the response is complete.
Inference is computationally expensive, especially with large models like GPT-4, LLaMA 3, or Mistral. Since these models are autoregressive, they generate one token at a time, making the process sequential and difficult to parallelize. Each token generation step depends on the previously generated tokens, adding to the latency.
Moreover, model size directly impacts inference cost. Larger models require more GPU memory and computing power, and they are slower to respond. For production use cases like real-time chat, content summarization, or retrieval-augmented generation (RAG), latency, throughput, and resource efficiency become critical.
In essence, LLM inference is where the rubber meets the road. It is the stage where model performance, infrastructure, and user expectations intersect, making optimization and scalability essential for real-world applications.
LLM Inference Techniques
Optimizing LLM inference is critical for delivering low-latency, cost-efficient, and scalable AI applications. Whether you're deploying a chatbot, powering a search assistant, or running a multi-tenant GenAI platform, the right techniques can drastically improve performance. Below are some of the most effective methods used to speed up and scale large language model inference in production environments.
Quantization
Quantization reduces the precision of model weights (e.g., from FP32 to INT8 or 4-bit), which decreases memory usage and speeds up computation. It enables large models to run on smaller or cheaper hardware. Methods like GPTQ and AWQ make this practical without major loss in accuracy. It's especially effective for GPU and edge inference.
KV Cache (Key-Value Caching)
Transformer models compute self-attention across all previous tokens at each step. KV caching stores these computations, so the model doesn’t have to recompute them every time a new token is generated. This significantly improves inference speed, especially for long prompts and conversations.
FlashAttention and PagedAttention
FlashAttention optimizes the attention mechanism by reducing memory overhead and enabling faster computation using CUDA-level tricks. PagedAttention (used in vLLM) manages key-value memory in blocks (pages), allowing for efficient handling of long sequences and batched inference with low latency.
Speculative Decoding
Speculative decoding uses a smaller model to predict multiple tokens in advance. The larger model then verifies or corrects these predictions in fewer passes. This parallelism reduces inference time while maintaining high response quality, making it suitable for real-time applications.
Model Compilation and Graph Optimization
Compiling models using tools like ONNX Runtime, TensorRT, or TorchScript creates static computation graphs that execute more efficiently. These frameworks optimize kernel launches, fuse operations, and reduce inference overhead, resulting in faster and more stable performance.
Efficient Batching and Token Streaming
Batching allows multiple inference requests to be served together, maximizing GPU utilization. Token streaming delivers outputs incrementally as they’re generated, improving perceived latency and responsiveness for users. Combined, they support real-time use cases at scale.
Benefits of LLM Inference Optimization
As organizations deploy LLMs in production, inference cost and latency quickly become limiting factors. Without optimization, even a moderately sized model can become prohibitively expensive or too slow to support real-time use cases. Applying the right inference optimization strategies can lead to substantial performance and business benefits.
Reduced Latency: Optimized inference drastically cuts down response time. Techniques like KV caching, batching, and quantization allow models to generate tokens faster. This enables smoother user experiences in applications like chatbots, virtual assistants, and generative tools, where responsiveness is key.
Lower Infrastructure Costs: Inference optimization helps reduce GPU memory usage and computational load, which directly translates to lower cloud costs. With quantized or compiled models, teams can serve the same workload using fewer or smaller instances, leading to improved ROI on compute resources.
Higher Throughput and Scalability: With optimized inference, you can handle more concurrent users or requests per second. This is particularly important for multi-tenant applications or platforms serving large-scale user bases. Batching, caching, and efficient memory management enable better utilization of GPUs, unlocking horizontal and vertical scalability.
Better User Experience: Fast and consistent responses help retain users and improve satisfaction. In use cases like search augmentation, live recommendations, or summarization, latency directly impacts how users perceive product quality. Optimization ensures that real-time interaction feels fluid and reliable.
Environmental Sustainability: Efficient inference also has sustainability benefits. Reducing compute cycles and energy use through optimization helps lower the environmental footprint of running LLMs, making GenAI applications more eco-conscious.
Optimizing LLM inference isn’t just about speed—it's a foundational step in building scalable, cost-effective, and high-quality AI applications.
Infrastructure Bottlenecks and Challenges
Deploying large language models (LLMs) in production is not just a software problem—it’s an infrastructure challenge. While model performance can be optimized at the algorithmic level, production-grade GenAI systems face a different set of hurdles that stem from hardware limitations, orchestration complexity, and scaling unpredictability.
- Optimization is meaningless without infrastructure readiness.
- Real-world LLM performance depends heavily on system design.
GPU Memory Constraints: LLMs often require tens of gigabytes of GPU memory to run efficiently. Hosting models like LLaMA 2 70B or Mistral 7B can easily exceed the capacity of a single GPU, necessitating model sharding or the use of high-end and expensive GPUs. Without optimization, memory becomes a bottleneck that limits batch size, slows inference, or forces expensive hardware choices.
- Large models can’t fit on standard GPUs without quantization or sharding.
- Memory bottlenecks directly affect latency and cost.
Load Spikes and Autoscaling: GenAI workloads are bursty. A sudden spike in traffic—say, during a product launch or viral moment—can overwhelm an unprepared system. Autoscaling GPU nodes is much slower than scaling traditional CPU workloads, especially in Kubernetes environments. Cold starts for LLM containers can take several seconds, adding to response latency when demand surges.
- Traditional autoscaling strategies are too slow for LLM workloads.
- Cold start latency can ruin real-time UX during spikes.
Multi-Tenant and Multi-Model Complexity: Running multiple LLMs or serving different tenants on the same infrastructure adds layers of complexity. You need to isolate workloads, manage fair resource allocation, and ensure that no single model starves others of GPU access. This often requires custom routing logic, API gateways, and fine-grained observability.
- Multi-tenant GenAI demands isolation and dynamic resource allocation.
- Improper routing can lead to noisy neighbor issues.
Network and IO Overheads: Inference latency is not only about model computing—it’s also about data movement. Tokenization, vector retrieval (in RAG systems), and API communication all contribute to end-to-end response times. Slow IO between components can negate even the most optimized model.
- Token-level latency adds up quickly in RAG and streaming setups.
- IO bottlenecks need monitoring and mitigation, not just faster models.
Deployment and Versioning Overhead: Iterating on LLM versions or switching between different model backends is painful without standardized pipelines. Model updates, rollback mechanisms, and compatibility issues introduce friction for engineering teams, especially when operating across environments (staging, prod, etc.).
- Releasing new model versions must be fast, safe, and observable.
- Manual versioning increases risk and slows iteration speed.
Serving LLMs in Production
Serving large language models in production requires thoughtful system design. It is not just about loading a model and exposing it through an API. Depending on the use case, such as real-time interaction, document processing, or knowledge retrieval, the architecture must balance latency, reliability, scalability, and cost-efficiency.
Choosing the Right Serving Framework
Choosing an inference engine is a foundational decision. Tools like vLLM, TGI (Text Generation Inference), and DeepSpeed inference each bring unique benefits. vLLM is built for performance at scale, using paged attention and KV caching to enable high-throughput, low-latency inference. It supports concurrent requests and is ideal for token streaming.
TGI offers an easier integration path, especially within the Hugging Face ecosystem. It supports advanced decoding strategies and built-in streaming, which makes it developer-friendly. DeepSpeed-Inference focuses on memory optimization and tensor parallelism, allowing large models to run even on constrained hardware.
- vLLM is best suited for high-performance, batched, and streamed inference.
- TGI and DeepSpeed-Inference provide simpler deployment and better memory control.
API Design and Streaming
Modern LLM applications need more than static responses. Streaming APIs improve user experience by delivering tokens in real time. This is critical for chatbots and assistants, where even a small delay can feel sluggish. Token-level streaming reduces perceived latency and makes interactions feel more natural.
Good API design also includes parameters like temperature, top_k, and max_tokens, which give developers control over model behavior. Providing metadata such as model version and latency stats helps with monitoring and debugging. Versioning and rate-limiting are also key for stability and scale.
- Streaming responses enhance user experience with faster feedback.
- Configurable and versioned APIs give flexibility and ensure reliable performance.
Observability and Monitoring
Inference systems often fail silently due to issues like slow generations, GPU throttling, or low cache hit rates. Without proper observability, teams are left guessing. Metrics such as prompt length, token latency, and GPU memory utilization must be tracked in real time to maintain performance.
Logging and tracing should happen at both request and token levels. This helps identify slow prompts, isolate infrastructure bottlenecks, and detect regressions early. Integrated monitoring tools allow teams to respond quickly and keep inference pipelines running smoothly.
- Token-level metrics are essential for debugging and optimization.
- Monitoring prevents silent failures and supports proactive incident response.
How TrueFoundry Scales LLM Inference
TrueFoundry enables efficient and scalable deployment of large language models (LLMs) through a Kubernetes-native infrastructure, optimized inference engines, and an enterprise-grade AI Gateway. This combination allows teams to deploy both open-source and proprietary models with high throughput, low latency, and full control over performance and cost.
At the foundation is a distributed GPU pool architecture, which spans across multiple cloud regions and providers. This setup ensures fault tolerance and reliability, especially when using spot instances. Incoming requests are funneled through a queuing system that decouples request intake from GPU availability, enabling asynchronous processing with only 5–10 ms added latency, negligible for most LLM use cases.
TrueFoundry supports optimized inference engines like:
- vLLM for continuous batching, page attention, and streaming.
- Text Generation Inference (TGI) for fast token throughput.
- Shared model volumes via EFS or similar, reducing redundant downloads during autoscaling.
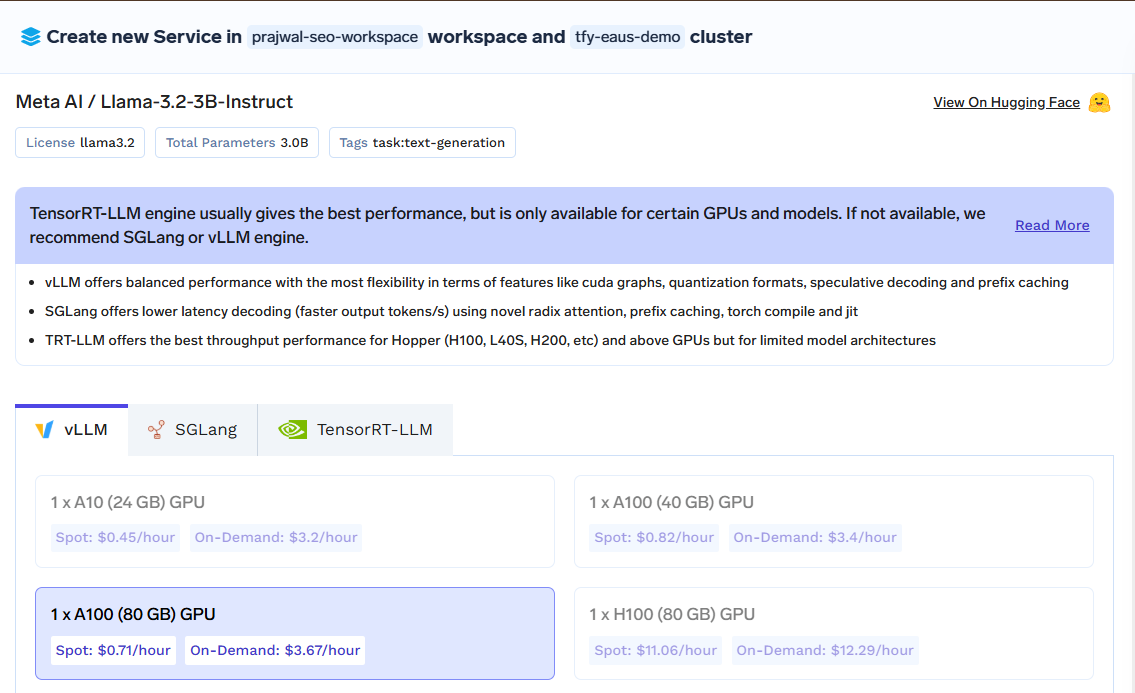
These engines significantly enhance token generation speed and multi-model hosting efficiency.
The AI Gateway acts as the central interface for both self-hosted and third-party LLMs. It offers:
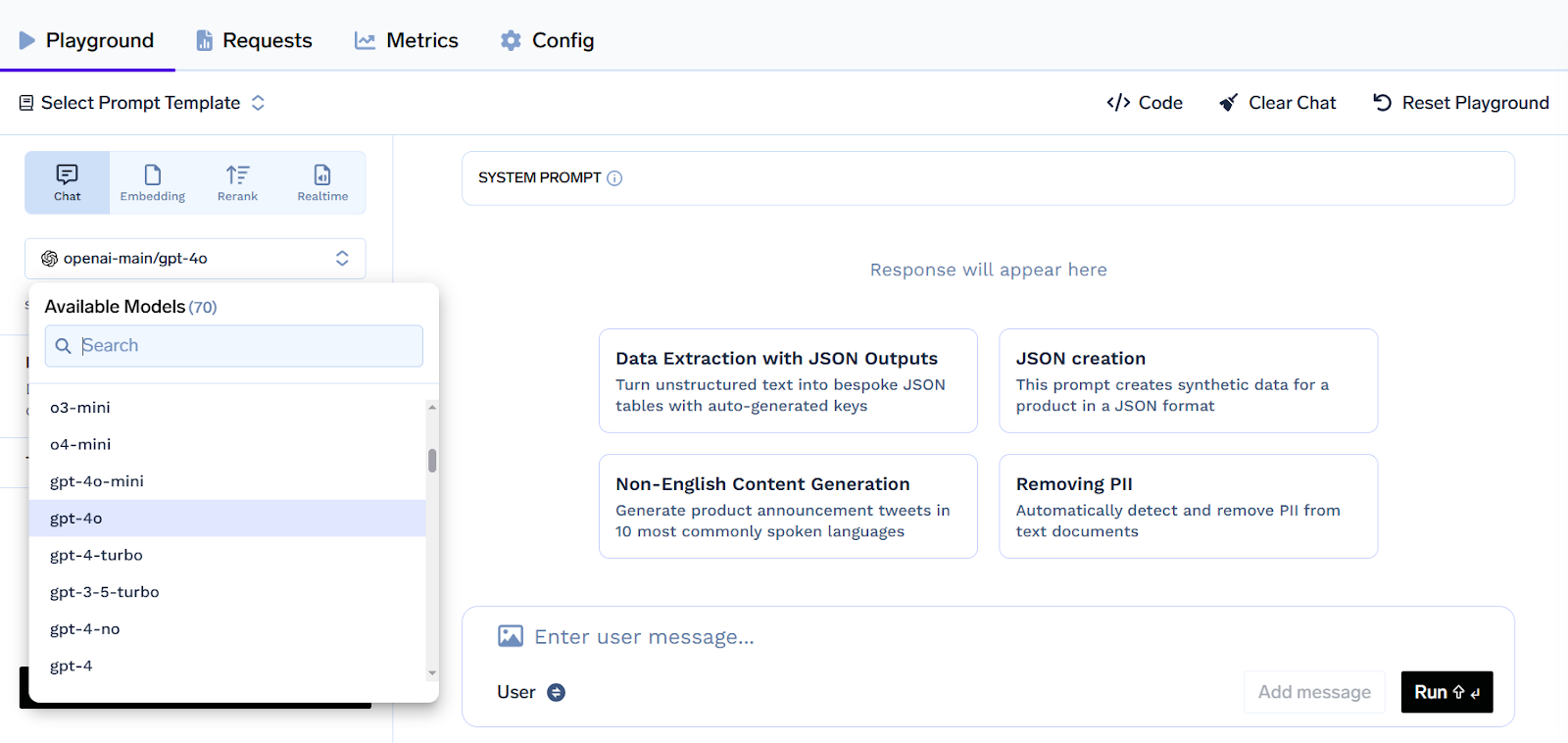
- OpenAI-compatible APIs for seamless integration
- Prompt templating and semantic caching to reduce repeat computation
- Intelligent fallback and multi-provider routing
- Rate limiting and authentication to protect model endpoints
TrueFoundry also features autoscaling based on real-time metrics like RPS (requests per second). Models are scaled up or down dynamically, ensuring resource efficiency during peak loads or idle times. Multi-region deployment and spot instance optimization further cut costs by 70–80%.
Finally, the platform includes robust observability across the LLM inference pipeline. Teams gain access to:
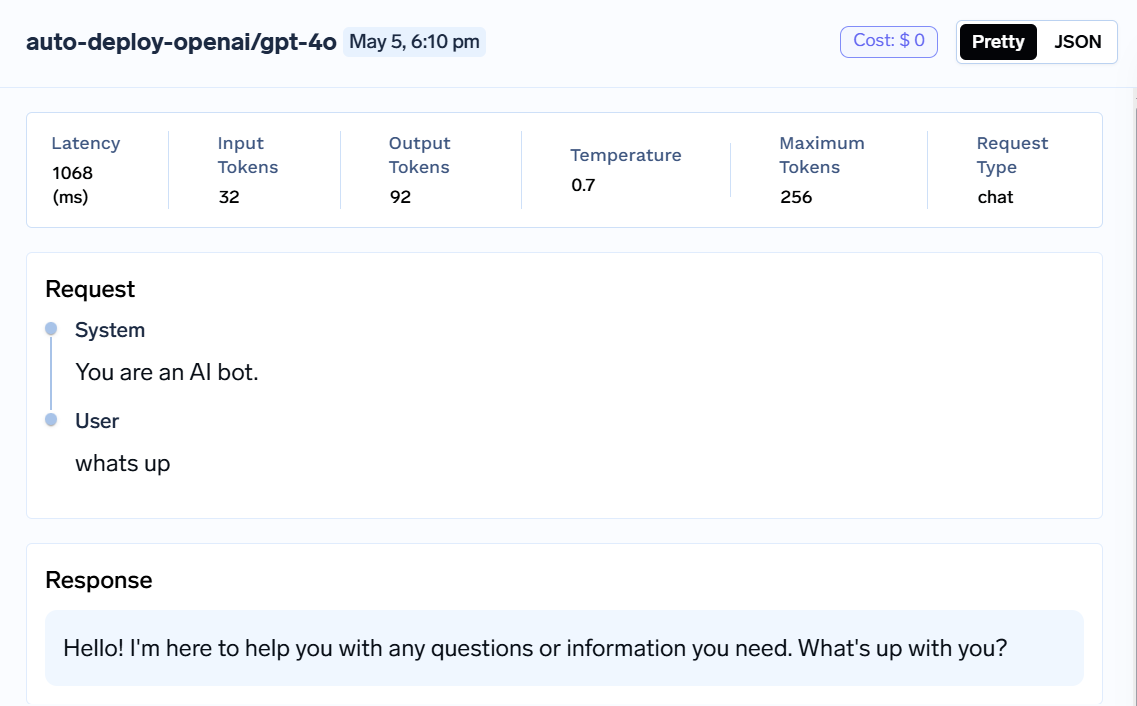
- Token usage and latency monitoring
- Prompt-level analytics
- Failure tracing and performance insights
By combining scalable infrastructure, optimized inference, and intelligent routing, TrueFoundry delivers an LLM serving stack built for production at scale, offering reliability, speed, and control without vendor lock-in.
Conclusion
As LLMs become central to modern AI applications, efficient and scalable inference is critical to delivering real-time, cost-effective user experiences. From quantization and KV caching to infrastructure-aware serving and observability, every layer of the inference stack must be optimized. However, building and managing this in-house can be complex and resource-intensive. TrueFoundry simplifies this process by providing a unified platform that abstracts infrastructure, automates serving, and enables production-grade GenAI at scale. Whether you’re deploying open-source models or building domain-specific assistants, TrueFoundry gives you the tools to run inference reliably, efficiently, and with full visibility into performance and cost.